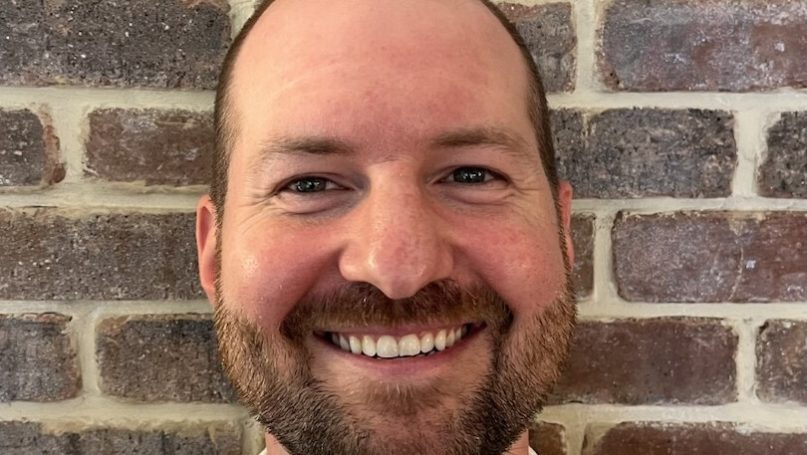
Andrew Halterman is an Assistant Professor in the Political Science Department at Michigan State University. He joined the department in 2022 after a year as a Faculty Fellow at NYU’s Center for Data Science. Andy earned his PhD from MIT in 2021. His research interests are primarily methodological, with a focus on automated text analysis and computational techniques. He has developed new techniques for extracting event data from text, geoparsing documents, and studying military operations. Substantively, he is interested in civil conflict, interstate war, and violence against civilians. His work has been supported by the National Science Foundation, Fulbright, the US Defense Department, the Political Instability Task Force, and the U.S. Holocaust Memorial Museum.
Where do you see the most exciting research/debates happening in your field?
I think many of the debates in security studies around threats, perception, and decision-making in conflict are still unresolved and likely to gain even more prominence as more of our attention is directed toward conventional military conflict after the invasion of Ukraine. For instance, I don’t think we have a good answer to the classic debate about when the Schellingian logic of deterrence is operating and when a Jervis-type spiral process is occurring. Understanding when and why each theory functions requires understanding how leaders perceive their opponents’ actions, and text is an excellent source of information for this. I’ve been very excited by the work that scholars like Marika Landau-Wells and Eric Min, among others, have been doing to use text to answer these types of questions.
How has the way you understand the world changed over time, and what (or who) prompted the most significant shifts in your thinking?
Before going to graduate school, I worked in DC on conflict forecasting and data analysis and that experience gave me a very pragmatic and applied approach to research. When you work on forecasting, you have to produce a concrete answer to a question, and you also have the benefit of finding out whether that answer was correct or not. Many of the big IR theories are useful lenses when trying to predict political processes, but each of them is only a partial account. The exercise of applying IR theories to prediction and reading the literature on forecasting and cognitive biases (e.g., Tetlock, Kahneman, Ulfelder) gave me a degree of flexibility and intellectual humility that has (I think) made me less overconfident than I was before graduate school. That work in DC also gave me a focus on developing tools and data for other researchers to use. It sparked my interest in becoming a methodologist alongside becoming an IR scholar, and my work now is motivated by understanding which tools and data will be useful for other applied researchers to use and trying to develop them.
How does natural language processing (NLP) help us better understand international relations and the world itself?
More than some other subfields, most of our data doesn’t arrive in an already structured format, so we have to be creative about how we produce data for us to use. Much of IR is concerned with understanding the thinking and behavior of elites. That’s nice from a natural language processing perspective, because elite decision-making and actions tend to get written down. Turning that text into usable data can be a difficult process, though. For many questions, the right way to work with text is qualitatively, which has a long tradition in IR. The other option is to use automated tools that turn text into structured data for us to analyze. That’s where NLP is very exciting, because it gives us a set of building blocks that we can modify and assemble into tools to extract the kinds of information we want from text.
In your work on NLP, you introduce the idea that an emerging third generation of text analysis draws on more advanced NLP methods. How is this generation different from those preceding it? How will these new techniques transform international relations?
I wrote the “three generations” piece as a way of understanding the history of text analysis in political science and to explain which techniques are appropriate for different projects. Scholars in political science, and IR specifically, have used keyword or dictionary techniques (the first generation) for over 30 years. Labeling documents by whether they contain a specific word or phrase (e.g., “human rights”, “police OR security services OR …”) is a simple, transparent, and powerful technique. Dictionary methods, however, struggle to identify more abstract concepts or to inductively learn the themes contained in documents.
Beginning about 15 years ago, researchers began using statistical techniques, including document classification and topic models, to categorize or group documents. These second generation models usually begin with a “bag-of-words” assumption, that the order of words in a document can be discarded and a document treated simply as a collection of words. These techniques, especially topic models, are very powerful and have enabled scholars in IR to study UN resolutions and speeches, intra-bureaucratic disagreements, censorship of news articles, and the writings of jihadi scholars. The bag-of-words assumption makes it easy to study the content or themes of documents but makes it difficult to extract specific pieces of information from within a document.
The techniques in the third generation of text analysis preserve word order and allow us to create different kinds of data from text. Advances in natural language processing allow us to identify people, organizations, and locations within a document, to understand the grammatical structure of a sentence, and increasingly, extract the phrase in a document that answers a question we provide. For IR, this is exciting because it allows us to extract descriptions of political events, such as battles or protests, or to identify relationships between entities, such as which people belong to which organization.
I want to be clear that the third generation isn’t necessarily better than the other two. I would still use a keyword technique to identify which documents talk about the United Nations, a topic model to identify the themes present in a Security Council debate, and only use a third generation technique if I wanted to identify the specific people alleged to be violating human rights in a Security Council resolution.
You have written about an automated machine learning system for geolocating political events in text. Could you explain this method and how it aids political science research?
My work on automated geolocation grew out of my research on event data in IR. Event data techniques turn descriptions of political events into a structured “who did what to whom, where and when?” format. The techniques for identifying the “where” were often lacking, however. My work on geolocation tries to address the two steps needed to associate an event with a place. First, for a place name mentioned in text, which geographic location is it referring to? Many place names are ambiguous–is a mention of “Tripoli” referring to the city in Lebanon or Libya? The problem is especially difficult for transliterated place names, where a single location can be spelled dozens of ways. My geoparser tries to resolve this ambiguity by using the context of the story, especially other place names in the text, to pick the correct geographic location and geographic coordinates for each place name mention. The second step is to associate events in a story with the place where they occur. A news story might have multiple events and multiple place names, thus linking each event to its location can be difficult. To address each of these problems, I collected a large amount of labelled data and trained a neural network to perform each step.
These techniques have applications beyond event data. For instance, a dataset I used in my work on Syria included a free-form text area with hand-entered place names. Converting the place names into geographic coordinates required handling ambiguous place names and many alternative transliterations. I think there are also promising applications for qualitative or archival work as well. For instance, a researcher who is interested in a specific area could geoparse a large corpus of text and pull out documents that mention places within their area of interest for further study.
You have examined existing theories of violence against civilians in the Syrian civil war using NLP. What new insights were gained through the use of NLP? Did they challenge or support existing theories on violence against civilians?
My project on violence against civilians in Syria involved collecting and combining several datasets on civilian victimization, territorial control, military offensives, and regime threat. I used NLP techniques in two ways to help construct the final dataset. First, the common link between each of the datasets was geographical. To identify the places where civilians were killed, I needed to convert the written place names in the raw dataset to geographic coordinates. To do so, I drew on the geolocation work I talked about above. I’ve also constructed a dataset of Syrian government military offensives using news text. To do so, I started with a dependency parse of the text, which is a technique from NLP that identifies the grammatical structure of a sentence. That information allowed me to identify who was attacking whom. A dictionary of terms identified when the actor was the Syrian military, and geoparsing techniques allowed me to associate each offensive with geographic coordinates.
Substantively, I found that a large proportion of civilian casualties occurred either in areas of ongoing military offensives or as a result of indiscriminate bombing behind front lines. This finding is in contrast to much of the literature on civilian victimization in civil war, which predicts greater violence in areas of partial control, rather than a fully contested area. However, much of the literature on violence against civilians is focused on the specific dynamics of insurgency, which are quite different from the conventional fighting in Syria. The findings match the conclusions of research on conventional (civil) war (e.g., Balcells, Downes), which reiterates the importance of applying theories within their scope.
Can the methods and insights used in relation to the Syrian civil war be applied to other instances of violence against civilians? What were the main challenges in carrying out this kind of analysis?
The civil war in Syria has unusually abundant data. The extensive work that individuals and NGOs did to document and report on the war meant that it was relatively easy to study using quantitative methods. I think future conflicts will have similar amounts of data available, making quantitative tools applicable. I think we’ve seen from the war in Ukraine how effective open-source intelligence analysts have been in understanding certain parts of the war. For instance, image and video geolocation techniques allow to precisely pinpoint where specific weapons systems are being used and unclassified overhead imagery can show us where military forces are located and assess battle damage. In the future, I’d like to see more of those techniques become automated and applied to social science research projects.
The major methodological issue in studying conflicts with automated techniques is the bias in reporting. There’s been discussion of researchers’ and journalists’ overreliance on Syrian opposition reporting in a way that potentially undercounts civilians killed by Syrian rebel groups. In my research, I came across a different issue, which was that Syrian government propaganda tended to be easier to scrape and was written in idiomatic English, while opposition reports were often written in less standard English. Many NLP tools are trained on highly edited English-language news articles, and I found that they often struggled to parse opposition reports, leading to potential bias.
On a personal level, the hardest part of working on this kind of project is the relentless tragedy of studying the war, and violence against civilians more specifically. Quantitative methods provide a kind of distance that qualitative researchers rarely have, but even so, every row in the dataset has the name, age, and cause of death of a civilian and it wears you down.
You have also proposed a novel way of quantitatively measuring regime threat. What was the process behind constructing this measure? What insights has it provided?
Many of the theories of violence against civilians in wartime argue that threats to the regime’s survival are a major determinant of whether the government will engage in violence against civilians. Measuring regime threat in a quantitative way is very difficult, though, and I couldn’t find a good existing measure of it. In the paper, I proposed using forecasts from the Good Judgement Project, a forecasting competition, about whether Assad would remain in power as a measure of regime threat. Forecasters were asked whether Assad would still be in power at some date in the future. By averaging the daily forecasts and normalizing for the time remaining before the cut-off date, I was able to generate a measure of perceived threat to regime survival. Although the forecasts were made by outsiders and thus not a direct measure of internal thinking in the regime, the GJP forecasts have been validated as a good predictions in general, so I believe this was a reasonable approach.
What are some of the challenges in making sure increasingly complex models remain verifiable and interpretable? Does this increasing complexity present an issue when using these models to support decision and policy making?
Machine learning models can be quite opaque. It is often impossible to determine why a model makes the decisions that it does. That said, I think that in some ways machine learning models can be easier to verify than many of the statistical tools we use in IR. For instance, there’s not really a way of knowing if the standard errors in your model are correct or whether you’ve included the right fixed effects in your model (see the large debate in the IR methods literature). In contrast, while we might not understand exactly why an NLP model identifies a particular set of words as, say, the target of an airstrike, we can easily verify that the model’s output is correct by returning to the source material. That process of verification is crucially important and can involve a great deal of work, but it’s quite possible to do.
That said, NLP tools are often just one step in a in a larger pipeline of data production and analysis. A typical NLP pipeline might take a document, preprocess it in some way, apply a couple of machine learning models to extract information from it, and then feed that data into a statistical model. Each of these steps will introduce error, bias, and uncertainty, and being able to propagate that uncertainty all the way through our final conclusions is one of the most important directions for current research in applied NLP.
What is the most important advice you could give to young scholars of International Politics?
I would have two pieces of advice for young scholars in IR. The first (and perhaps I’m biased as a methodologist), would be to take as many quantitative methods courses in grad school as possible. For most of us, the quantitative skills we learn in graduate school are the skills that carry us for our entire career and building a library of techniques early on will serve us well. Learning these quantitative techniques on our own is very difficult, and when we have good instructors for methods, we should avail ourselves of their expertise. Alternative careers outside of academia, in industry or government, also really prize these skills and researchers in IR with strong quantitative backgrounds will have a large set of career opportunities to choose from.
The other piece of advice I would have is to work on what you’re excited about and not try too hard to optimize for what’s interesting to other people. Grad school takes up a large portion of our lives and it’s too many years to spend working on something that you don’t enjoy, just for the possibility of some later payout. Or, to put it in economics jargon, I think graduate school has to be at least partially a consumption good and not purely an investment. So, find a group of researchers you enjoy collaborating with and work on the problems you’re excited about.